Why Silicon Valley should take ag tech more seriously
Arian Aghajanzadeh for GreenBiz: The global agricultural sector consumes 2,600 terrawatt-hours of energy and emits 5.3 billion metric tons of carbon dioxide equivalent every year. That is roughly equal to the emissions from over 1 billion passenger vehicles driven for one year (more than the number of passenger vehicles currently in operation worldwide) or the emissions from 1,300 coal-fired power plants in one year (the same number of coal-fired power plants operating in the United States).
Given that data, it is clear that we cannot combat climate change without considering the agricultural sector more thoughtfully.
As smart and connected devices have become ubiquitous in our daily lives — and the disciplines of data science and artificial intelligence have given us the tools to solve ever more complex problems — the agricultural sector seems to have benefited very little from such advancements.
A 2015 report by McKinsey & Company stated that agriculture and hunting remain the least-digitized industries in the United States. However, farmers long have sought cost-effective tools to increase the efficiency of their fields. Sensors that measure air and soil, livestock biometrics and automated systems that use the internet of things (IoT) to control irrigation are just some tools already available. Precision equipment, geo-positioning systems, big data, unmanned aerial vehicles, drones and even robotics are also leaving their mark on farming. Full Article:
Comments (0)
This post does not have any comments. Be the first to leave a comment below.
Featured Product
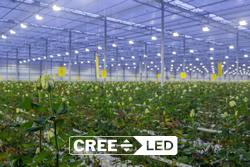