Even if we had perfect weather data everywhere all the time, there are many more uncertainties in a lot of the weather-driven agronomic models that attempt to predict crop stage, disease pressure, and crop performance
How Machine Learning Plus Weather Information Can Help Us Feed The World
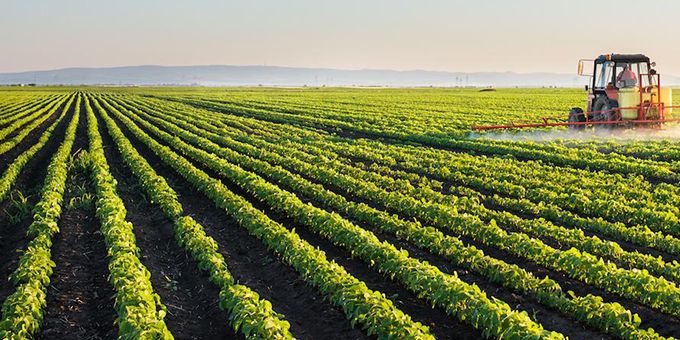
Brent Shaw | Iteris, Inc.
The world of technology-based solutions has been increasingly flooded with buzzwords like “analytics”, “big data”, and “Internet of Things”. In the past few years, the availability of cost-effective, large-scale cloud computing and open-source scientific programming libraries has led to the proliferation of “machine learning”, “artificial intelligence”, and similarly named services that essentially promise to turn your data into actionable information or insights.
Having spent most of my career working with large, diverse meteorological datasets and developing software for automated weather forecasting and decision support, I am always intrigued by new ways of applying the latest technology to my domain. The challenges in precision agriculture and their intersection with weather and soil conditions are what led me into where I am today. I am using this month’s column to shed some insight on where I see machine learning (ML) techniques bringing the most value to weather-sensitive operations in agriculture.
Solving the Right Problems with the Right Tools
Unfortunately, what I see happening are lots of enthusiastic and brilliant data scientists, armed with powerful tools and access to amazing computational resources, charging off to solve problems for which they have little or no practical knowledge, believing that given enough data they can solve any problem in any data-rich domain. I appreciate that enthusiasm, and welcome the help in meteorology and agriculture, but want to make sure we attack the right challenges with the right tools, without expending much effort reinventing slightly better wheels while promising flying cars.
ML systems are becoming high-powered jackhammers in the buzz of the marketplace. And when then the one tool in your belt is a hammer…well, I don’t need to pound that analogy in again. As we try to leverage technology in agriculture to feed the world, the last thing we need are disappointing results in a world of high expectation that lead to distrust and slower adoption of truly useful solutions. But jackhammers are important when they are needed and have many applications – as is the case with ML.
You might be surprised to know that the use of ML in meteorology is not new at all. Weather really is the original “big data” problem before it existed as a buzzword. Operational forecast services in both government and the private sector have employed various ML methods for decades as part of data analysis and automated prediction, but not as a standalone tool in the sense that most people might think of, whereby a bunch of historical data gets put into a magical, ML “black box” resulting in a capability to simply forecast the future from past data.
In a previous article I explained the various forecast techniques and their applicability to different time scales of prediction. None of those techniques are foundationally ML based, but most of them employ ML as part of the process, whether it be to find and remove erroneous input data, adjust the final output of a physical model based on past performance, or to optimally combine multiple pieces of information into better weather intelligence.
For example, neural networks have been employed to allow improved quality control of raw radar data, helping to remove false echoes that a human analyst can readily recognize but is much more difficult to do in an automated fashion. These false echoes can lead to bad precipitation estimates.
As another example, our company has long used an internally developed multi-model ensemble forecast system where each individual model is compared with recent ground truth data, corrected for biases and then blended with other models using a dynamic weighting scheme to arrive at the best forecast. This helps us produce very reliable, probabilistic precipitation forecasts that are suitable for objective decision making.
As mentioned in my earlier article, the physics-based numerical weather prediction models have been, and for the foreseeable future will continue to be, the primary tool for forecasting the next few hours to weeks of weather. Any attempt to use pure ML techniques as an alternative, rather than a supplement, to these models would be an inefficient use of talent and resources, in my opinion. ML techniques may offer more promise in longer range, such as seasonal and longer outlooks, but I remain skeptical of the value of those for real-world, agricultural decision making, and there may be a practical limit in forecasting regardless of technique, ML or otherwise.
Where Machine Learning Adds Value
Where I think we can reap large benefits from ML is in the use of diagnostic and predictive analytics of weather impacts. That is, the response in grower behavior, plant or product performance, or pathogens that is likely related to multiple elements of the weather and/or other inputs, but where those interdependencies are not fully understood.
Even if we had perfect weather data everywhere all the time, there are many more uncertainties in a lot of the weather-driven agronomic models that attempt to predict crop stage, disease pressure, and crop performance, and variability due to regional and genetic differences make phonological modeling difficult. Additionally, things that have behavioral inputs, such as a producer’s response to certain conditions, or migratory patterns of pests, cannot be simply turned into equations. In these cases, ML offers high potential to add tremendous value.
For example, one of my colleagues has been working on applying artificial intelligence to crop modeling. Using a neural network method to combine a large dataset of crop stage observations with high-quality, temporally and spatially consistent weather and soil information for the field, he demonstrated a capability to generate a predictive crop model for use in stage-dependent field operations.
Another example where my colleagues have applied ML techniques is in determining how accessible a field is for equipment to help producers make informed decisions about moving equipment when managing geographically dispersed field. The challenge in this case is that what is considered workable by one grower with his particular equipment can be much different than another, even in the same area, and the system may not know anything about the grower’s behavior or equipment. Thus, a method of accepting feedback on the operator’s assessment of field conditions is used in a ML system to train the model to the particular operator’s preferences on a field-by-field basis over time, continually improving the automated assessments.
Those examples were made enabled by two things. First, having a team of people, experts in their particular relevant field, working closely with a data science expert is critical. Each expert brings something valuable to the table, learning from each other about the nuances of the operational problems, the kinds of data (and their nuances) available for use, and insights into where to focus efforts. I cannot emphasize this enough: a cross-disciplinary approach, bringing relevant domain expertise into any technology solution development is key.
Second, the availability of usable data for training the ML techniques is critical. This may be the largest challenge we face in precision agriculture, as we continue to struggle with standardization of data exchange protocols and a generalized fear of sharing. We have to work together to protect data in a way that keeps the owner comfortable while benefiting the industry as a whole. This is all possible today, but we have to overcome barriers that have only been strengthened by recent, highly publicized abuses of end user information collected by consumer applications.
When it comes to mitigating losses and enhancing production through better weather and soil analytics, the best solutions will come from teams of atmospheric, agronomic, soil, and data scientists working with tech-savvy agribusinesses and producers. It’s important to not get distracted by buzzwords, and instead make sure the right people are using the right tools to solve the right problems, and then feel good about sharing relevant data that can help us collectively feed the world more efficiently.
About Brent Shaw
Brent Shaw is vice president of weather content and customer success at Iteris, and is a leader in applied quantitative meteorology.
The content & opinions in this article are the author’s and do not necessarily represent the views of AgriTechTomorrow
Comments (0)
This post does not have any comments. Be the first to leave a comment below.
Featured Product
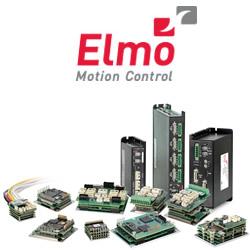