Agriculture Needs to Access Big Data to Enhance Research, New Paper Suggests
"The next generation of agricultural problem solving will require big science and linkages forged across data sets and disciplines," the new Council for Agricultural Science and Technology paper argues.
Utilizing big data will advance the field of agriculture as long as its researchers are willing to embrace change and adopt collaborative strategies, a new CAST paper suggests.
The commentary argues that "information, including all data, collected from publicly funded scientific activities belongs to the public and should be freely available and usable."
Much of the data captured by organizations conducting research is not shared with outside scientific communities, and incidental data is often kept private. This impedes scientists' abilities to generate richer analyses from the entire data set and apply it beyond its primary purpose.
"If we really want to democratize access to science, facilitate innovation, and address grand challenges to our current food systems, we need to develop mechanisms to incentivize an infrastructure to support open data," says Sylvie Brouder, professor of agronomy at Purdue University and the paper's task force chair.
The paper also outlines strategies academic institutions and their partners should consider to enable more data-driven research. One strategy involves producing a sustainable open-source data network that allows scientists easier access to previously inaccessible data sets.
However, the new approach comes with a learning curve. Researchers will need to collaborate with data scientists and participate in educational opportunities to better understand the process of working with an unfamiliar source of information.
"I was never taught how to be a good steward of data; it is only recently that I became aware of how much of an impediment this was to delivering research with added value and impact," Brouder says. "Not everyone needs to become a data scientist, but we need to enhance data literacy across most of the agricultural disciplines."
The paper is now available to download on CAST's website.
Task Force Authors
Sylvie Brouder (chair), Purdue University
Alison Eagle, Environmental Defense Fund, Raleigh, North Carolina
Naomi K. Fukagawa, USDA-ARS, Beltsville, Maryland
John McNamara, Washington State University (retired)
Seth Murray, Texas A&M University
Cynthia Parr, USDA-ARS, Beltsville, Maryland
Nicolas Tremblay, Agriculture and Agri-Food Canada, St-Jean-sur-Richelieu, Canada
Featured Product
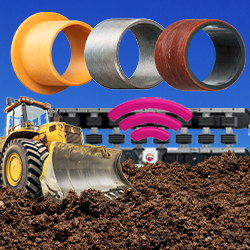
igus® - Free heavy-duty plastic bearings sample box
The iglide® heavy-duty sample box provides a selection of five unique iglide bearings, each suitable for use in heavy-duty equipment due to their self-lubricating, dirt-resistant properties. Each bearing material boasts unique benefits and is best suited for different application conditions, though each can withstand surface pressures of at least 11,603 psi at 68°F.