Through this new technology, robots will run through strawberry greenhouses in the north of England, gathering video footage that gets analyzed by AI as the robot moves along.
How the University of Lincoln Uses AI to Grow the UK’s Millions of Strawberries to Perfect Ripeness
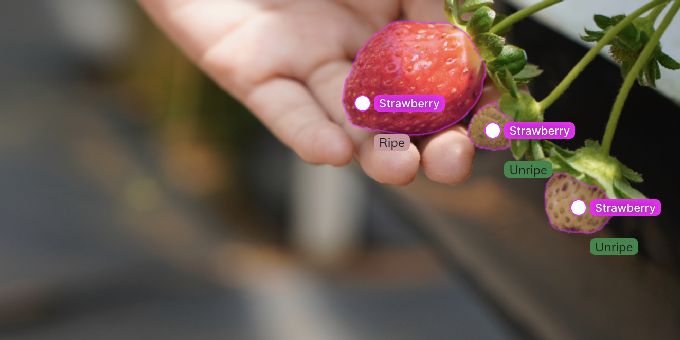
Article from | V7
Biting into the perfect strawberry and savouring its sweetness mostly depends on whether it was picked at the right time.
Picking labour accounts for 40% of horticulture production costs, and much of the remaining costs derive from berries being picked at the wrong time, leading to spoilage, or sour under-ripeness.
The Lincoln Institute for Agri-Food Technology (LIAT) at the University of Lincoln partnered with London-based AI data engine V7 to enable robots to analyze millions of strawberries throughout their growth cycle, monitoring weather conditions and berry appearance to predict the perfect harvest date for each plant.
“The UK produces 120,000 tonnes of strawberries each year, with a retail value of £659 million,” said project founder Raymond Kirk. “Predicting the timing and yield of strawberries is critical for the industry – but extremely difficult to do accurately.”
That’s the equivalent of roughly 15 billion strawberries picked in the UK alone. A large part of this is picked at the wrong time and goes to waste.
Through this new technology, robots will run through strawberry greenhouses in the north of England, gathering video footage that gets analyzed by AI as the robot moves along. The AI is able to spot each strawberry, including the unripe green ones hidden amongst the foliage, and every berry’s location. This has allowed the LIAT team to predict the day of perfect ripeness, and the total number of punnets a greenhouse might create 6 weeks ahead of current forecasting systems.
The technology is about to be rolled out as a commercial spinoff from the university to strengthen the UK’s horticulture industry, which is recovering from the impact of leaving the European Union that caused a shortage of skilled pickers.
The Humans who Train AI
Artificial intelligence emulates human intelligence by learning from many, many examples. For a robot to learn to detect the perfect strawberry across all angles, lighting conditions, berry varieties, and maturity states, it must learn from a lot of human knowledge, but how?
This “knowledge” is called training data. The process of humans “teaching” AI by producing training data is known as annotation. This involves drawing boxes or shapes around objects on software such as V7’s and then classifying the item, such as a strawberry of a certain variety or ripeness level. The more training data, the better the algorithm.
Once enough training data has been collected, AI models can be trained that ingest this data and test themselves against un-seen images. What has set V7’s technology apart is the ability for humans and AI models to work side-by-side on annotation challenges.
Reliability is also a challenge. Whilst most engineering disciplines have predictable failures, AI models can’t be “looked into” to analyze what they don’t know. This is why understanding an AI’s training data is so crucial, it acts as the repository of that AI’s total knowledge. Once an AI is “trained”, this knowledge is compressed into a neural network’s millions of values, which aren’t readable by humans.
How it was built
LIAT’s wheeled robot would capture imagery of the strawberries and load them onto the V7 platform, where humans would begin distinguishing different strawberry types. After a few hundred examples are provided, the teaching is sped-up as early AI models begin pre-completing training data to cover cases where their confidence is high.
"We needed a tool that could do annotating and data versioning because we distribute our tools to farms, and we need to make sure that they have the same version of data for the same models. V7 met our needs.” Raymond Kirk, LIAT project lead
The annotation process is often one of the bottlenecks of AI’s commercialization, as no company wants to dedicate their internal machine learning resources to clicking repetitively on images to train AI. V7 facilitates the sourcing of teams of humans that can kickstart the process, creating an assembly-line workflow for adding human knowledge to training images.
“Labeling in-house is a nightmare. It’s much easier just to upload data to V7 and outsource the whole annotation process hasslefree.” Raymond Kirk, LIAT project lead
User experience design was also mentioned as a deciding factor on the tools to build AI, as V7 enabled non-technical users to create training samples smoothly, whilst integrating with the wider ML-Ops environment that AI engineers need.
AI in Agriculture today
Artificial intelligence’s use in agriculture isn’t restricted to fruit picking. As one of the fastest-growing industries leveraging AI, it boasts several use cases including the monitoring of farm animals’ health, analyzing crops from satellite imagery, or detecting pests through cameras deployed on fields.
LIAT’s group itself is working on research to extend this technology to other berries, as well as mushrooms and other vegetables for yield estimation and robotic picking.
LIAT’s Robot today
The image data from LIAT’s robot-mounted cameras is now used to measure individual fruit numbers, along with the weight and maturity state of millions of berries each day.
This data is combined with weather forecasts in algorithms to predict yield six weeks ahead of existing systems. The effect of annotating using V7’s AI-assisted approach also helped increase the algorithm accuracy from an 85% human-only baseline, to 95%.
View Case Study: How CattleEye Uses V7 to Develop AI Models 10x Faster
About V7
V7 is a London-based AI startup founded in 2018. It develops an online training data platform to automate the process of AI data annotation, enabling AI-first companies to programmatically add labels to images or video. Its customers include GE, Boston Scientific, Fujifilm, and Miele. V7’s mission is to enable any business to create AI engines that automatically solve any task by emulating human actions performed on their platform.
About LIAT
LIAT’s mission is the development of technologies that add value or solve challenges across the food chain. In this project, they focused on the horticulture sector—starting with a small strawberry farm located near Riseholme Campus. The team uses vehiclemounted cameras to identify and count individual fruits, estimate their weight and maturity state. This data is used to develop AI algorithms for strawberry yield forecasting.
The content & opinions in this article are the author’s and do not necessarily represent the views of AgriTechTomorrow
Comments (0)
This post does not have any comments. Be the first to leave a comment below.
Featured Product
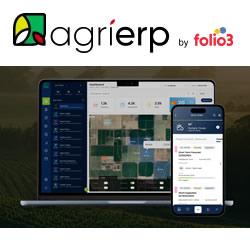