A coalition of extension pathologists from five midwest universities and Ontario Ministry of Agriculture are participating in a study of SDS outbreaks to determine how to predict where they are most likely to occur and the severity of their occurrence.
Developing Big-Data Models of Soybean SDS with Agmatix
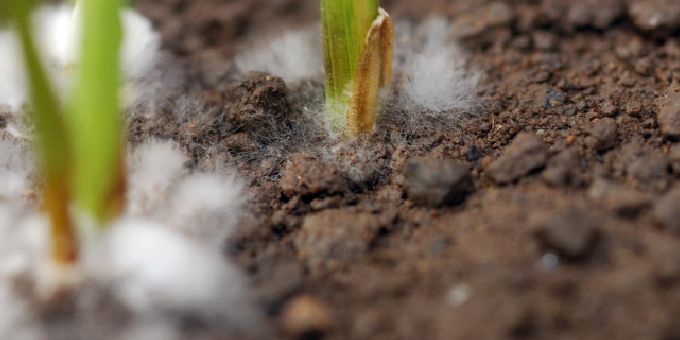
Case Study from | Agmatix
During the years from 2015 to 2019, soybean diseases were responsible for losses of around 9% of the production potential in the U.S., equals to $3.8 billions annually (Bi et al. 2020).
Soybeans (Glycine max) are the primary host for Fusarium virguliforme, a soil-borne pathogen responsible for soybean Sudden Death Syndrome (SDS), which has become a leading cause of the decline in soybean crop yield in North America. First seen about 40 years ago, SDS is currently detected in most soybean-growing areas in the United States and other main soybean production areas (i.e. Argentina, Brazil), and continues to spread.
Challenge
To prevent the continued decline in soybean yield, farmers must be able to predict the location of SDS outbreaks and their severity. Many times, when SDS symptoms appear, it may already be too late to stop the progression of the disease. This points to a need for an agricultural predictive modeling tool that can be used to explore relationships among key factors contributing to the spread of SDS and facilitate the development of data-driven agricultural solutions. This task is complicated, however, by the large number of factors that must be taken into consideration, including sanitation; weather conditions; patterns of irrigation and crop rotation (which can affect soil infection); history of disease occurrence in the field, planting time, spacing, and overlapping crops; the plant’s natural resistance to fungi; and use of fungicides. Thus, soybean farmers require agronomic analysis software that can handle large amounts of data on soybeans crops and their management.
The Agmatix Solution
A coalition of extension pathologists from five midwest universities (Iowa State University, Michigan State University, Purdue University, University of Illinois, and University of Wisconsin-Madison) and Ontario Ministry of Agriculture are participating in a study of SDS outbreaks to determine how to predict where they are most likely to occur and the severity of their occurrence. Their findings may allow soybean farmers to make optimal decisions regarding the time for planting, use of cultivars, population density, and other factors to prevent this disease.
This study was based on data derived from six studies of a total of 90 SDS field trials carried out over 5 years in six locations in the United States (5) and Canada (1). SDS field observations were augmented with relevant management data and weekly weather information, and served as input to a ML model – XGBoost, an ensemble of decision trees. The challenge of standardizing the terminology, measurements, etc., of such a large amount of data was met using the Agmatix protocol GUARDS (Global Universal Agronomic Data Standard), an agronomy data standardization tool in which trial-specific definitions and data measurements are standardized and anomalies that could skew analytical results are identified. Thus, they were able to transform trial-related data into a harmonized and Interoperable dataset.
Results
The researchers were able to predict absolute disease severity with a mean absolute error of 7 (FDX, the SDS severity index, is unit-less). When the data were divided into 3 classes – no disease, moderate disease, or severe disease – the model was able to predict the correct class with an overall accuracy of 78%, overall recall or 0.79, and an overall F1 score (a classification efficiency measure accounting for both precision and recall) of 0.71. These values indicate an overall satisfying classification model. Model sensitivity analysis found that SDS severity is affected by several management and climatic factors, such as cultiver genetics, timing and type of applied fongicide, and (among others), rainfall during specific crop growth stages.
The Conclusion
The preliminary analysis demonstrated the power of data standardization, and the coupling of field agronomic data with weather information, to adequately predict the occurrence of a complicated crop disease. Given more data, this collaboration could be transformed into an agricultural predictive modeling tool that soybean farmers can use to manage and reduce the risk of SDS occurrence in their fields.
The content & opinions in this article are the author’s and do not necessarily represent the views of AgriTechTomorrow
Comments (0)
This post does not have any comments. Be the first to leave a comment below.
Featured Product
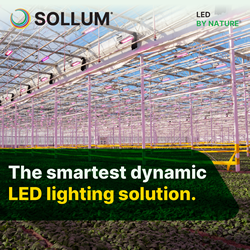