Although they promise streamlined farming operations, higher yields and greater costs savings for growers, how much of AI and machine learning in agriculture today is more hype than reality?
Artificial Intelligence And Machine Learning In Agriculture Today
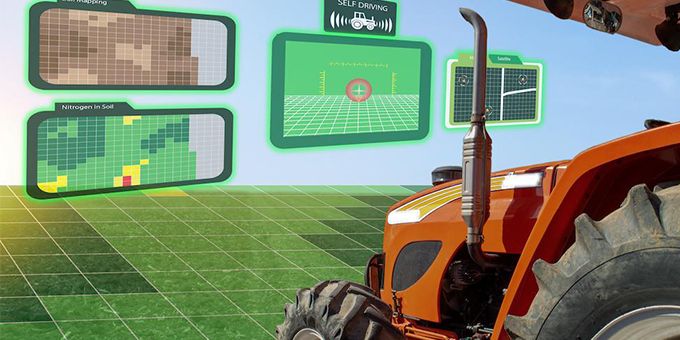
Rebecca Sakayeda | Iteris, Inc.
Reprinted with permission from Iteris, Inc.:
Artificial Intelligence (AI) and machine learning are terms garnering lots of attention these days, in many industries, including agriculture. And although they promise streamlined farming operations, higher yields and greater costs savings for growers, how much of AI and machine learning in agriculture today is more hype than reality?
We posed these questions to Jim Chambers, senior vice president and general manager of Iteris’ Agriculture and Weather Analytics division:
How are AI and machine learning used in agriculture today?
AI and machine learning are used in a number of agricultural applications today. Examples include yield prediction algorithms based on weather and historical yield data; image recognition algorithms to detect pest and diseases in plants; and robotics to harvest different types of specialty crops. So, it’s a growing technology that has increasingly important applications to improve agricultural processes.
What are some of the challenges and roadblocks to implementing AI and machine learning in agriculture?
There are a few. First, in agriculture, it’s important to understand that machine learning, like other technologies, is a part of a process, not a stand-alone solution. AI must provide practical applications that align with existing agricultural operations.
Next, machine learning requires lots of data around user or producer inputs, or patterns such as the migration of pests for example. Large gaps in data collection, preparation, and benchmarking capabilities exist today. This makes modeling a challenge.
Part of the “hype” of AI and machine learning stems from the fact that start-ups – many with little or no agriculture experience or background – use lean methods to get their technologies to market quickly, without understanding implications of the gaps in data. In addition, farmers are cautious to adopt new technologies because they don’t want to risk unproven technologies on their farms.
Is Iteris using AI and machine learning today? And if so, how?
Because of our team’s deep agronomic, atmospheric, soil and data science expertise, we’ve taken a very practical approach to AI and machine learning. Our applications are based on advanced diagnostic and predictive analytics and ground-truthed agronomic models. Our technology was built, right from the start, for machine learning. We focus on real-world challenges faced by agribusinesses and their grower customers.
Our AI and machine learnings applications include:
- Irrigation and Water Management: Understanding how soil moisture responds to irrigation events with different crops, soils, environmental conditions. When tied to an irrigation control system, this information can automatically implement control strategies that help minimize water usage, manage nutrient losses, or achieve more desirable or uniform soil moisture throughout the field.
- Crop Modeling: We apply artificial intelligence to crop modeling, generating a predictive crop model for use in stage-dependent field operations. Combined with producer feedback on crop growth stages, the model re-calibrates and refines itself. Where no phenological models have yet been developed, ClearAg employs a Neural Network technique to develop predictive models from past observed crop data by combining with our extensive historical weather data.
- Field Accessibility: We apply machine learning techniques to determine how accessible a field is for equipment to help producers make informed decisions for operational efficiency without knowing the growers’ specific equipment or operational practices.
- Harvest Decision Support: Crop moisture content models are developed using machine learning to combine observed moisture values with historical weather, and then applied in predictive mode.
- Weather Forecast Model Calibration: The individual numerical weather models feeding our proprietary ensemble forecast system are auto-calibrated using past performance against ground truth data and dynamically weighted.
The content & opinions in this article are the author’s and do not necessarily represent the views of AgriTechTomorrow
Comments (0)
This post does not have any comments. Be the first to leave a comment below.
Featured Product
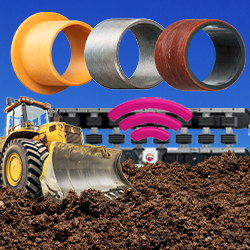