With Agrilyst, growers can understand which of their crops are the highest performers based on yield, growth rate, or both and run production scenarios with higher performers having more space allocation. These recommendations help growers optimize the space on their farm and drive significantly higher revenues.
Beyond the Hype: AI in Agtech
Allison Kopf | Agrilyst
Reprinted with permission from Agrilyst's blog:
Google “AI in agriculture” right now and you’ll see tons of articles about startups raising money, established big players wading into the space, and the occasional bovine artificial insemination article (we’re in ag after all).
AI is hot. And rightfully so. Agriculture is a low-margin, labor intensive industry. Technology can help farmers increase margins and decrease operational risk (crop loss, disease, food safety, pests, etc.). AI becomes increasingly exciting as we think about feeding our growing global population in more sustainable ways.
With a lot of attention, comes a lot of hype. We’ve been focused on using data to automate farming workflow since early 2015. Building good algorithms isn’t easy. You have to collect the right data points, reach data saturation, understand how the past influences the future, and know what your user’s primary objectives are throughout their workflow.
The stages of AI from least automated to fully automated.
Agrilyst has always been focused on one thing: helping growers increase profits significantly.
To do this, we have a few theses:
Data related to one function alone isn’t going to move the needle. I think it’s great that there are companies improving sensing technologies for smarter climate control. But better climate control isn’t going to push farms past profitability. I think it’s great that companies are creating algorithms to predict disease in crops using imaging. But imaging without climate and nutrient data isn’t going to be the most accurate predictor.
Indoor farming is the best application for AI in ag. There are over 6 million greenhouse growers around the world growing shorter cycle crops than commodity crops. That’s a massive data set. If a corn farmer has 40 chances in their lifetime to apply data to increase yields, an indoor lettuce grower might have 40 chances in 40 days if they’re planting and harvesting on a daily basis. It’s a faster and more consistent data set. Plus because growers are controlling their environment the controllable inputs are variables in an equation as opposed to uncontrollable factors.
Always build value. Our customers are farmers. They’re not cheap. They’re not tech-averse. They’re building a business on incredibly low margins. So technology has to work for them. No exceptions. AI for AI’s sake is not going to work in agriculture. Everything we build serves a specific value-added purpose for our customers — always.
How does Agrilyst collect data?
Agrilyst is a workflow management system. We’re focused on using data to automate the non-mechanical processes on a farm. Processes like production planning, crop scheduling, quality optimization, risk management (disease, pest, crop success), labor planning, sales, and inventory management all fall into our domain. We don’t make hardware and we’re not focused on automating processes that can be replaced by machines, like seeding, harvesting, processing, and packaging.
Our software is a critical part of running daily operations on a farm. Growers use Agrilyst to track crops from seed to harvest, store yield data, track pests, record nutrient metrics, and more. All of this user-generated data makes up the core of our data set.
Agrilyst’s data flywheel
We also have an open API to connect to any device a grower has on their farm. Growers who have sensors, climate control systems, nutrient dosing systems, or connected lighting can connect to Agrilyst through the API for real-time data collection.
All of this data combines to build a proprietary network of data that strengthens as it interacts.
Descriptive Analytics — What happened?
The first step in building an AI-driven workflow is digitizing all of the manual data collection processes our users used to write down on paper (thesis point: data related to one function alone isn’t going to move the needle).
That all starts with growth cycles in Agrilyst. Production planning used to take hours out of a grower’s day. Now growers drag and drop stages of a plant’s lifecycle into a template and then tell the system how often the template should repeat. This saves growers an average of 1 hour and 15 mins per week in manual work (thesis point: always build value).
We use this data as the foundation for showing growers what’s going on in their farm. In Agrilyst’s dashboard and with reporting tools, growers can drill down into their operational data to understand what is going on at all times.
Visual of a grower’s water metrics.
Diagnostic Analytics — Why did it happen?
The next step is using the foundational data to understand why something happened. This is what drives the training for algorithms and helps us get to the next step: predicting what’s going to happen.
Let’s say a grower sees a decrease in yield. We want to know why that happened. If the pH for a lettuce crop falls beyond the accepted range of 6–6.5, certain nutrients become unavailable to the plants. A common thing for our growers to analyze is pH trends against yield to see if this is the root cause for changes in yield.
Predictive Analytics — What could happen?
Now comes the fun stuff. At data saturation, roughly a few million harvest data points for us, our algorithms start to look forward to the future. How are crops going to perform? What factors are going to impact performance the most? How is space going to be utilized months out in the future? Do we have enough space to meet sales demands in the winter and summer? All of these questions are able to be answered once a system understands how the plants grow and all of the variables that affect that growth. Our most utilized algorithm is our yield forecast, which helps growers understand their expected harvest yield 30-days in advance at 90% accuracy.
Prescriptive Analytics — What should happen?
All of this leads to providing growers with recommendations. If I break down production planning into it’s variable inputs, you have:
- Crop selection (what crops are you growing?)
- Crop growth rate (how fast does it grow?)
- Growing area (how much space do I allocate to my crop?)
- Climate
- Nutrients
- Customer needs (how much am I selling, to whom, when?)
Once you have those pieces, you can create a production plan pretty easily. If I know Whole Foods needs 100 pounds of kale in 30 days and that kale in my system takes about 30 days to grow at roughly 1 pound per square foot, I’ll seed enough to fill 100 square feet of space today. And that’s how production planning happens today (without tech).
With Agrilyst, growers can understand which of their crops are the highest performers based on yield, growth rate, or both and run production scenarios with higher performers having more space allocation. These recommendations help growers optimize the space on their farm and drive significantly higher revenues.
Cognitive Analytics — Make it happen.
Here’s where the real magic happens. Because Agrilyst is focused on automating all processes that rely on human decision-making and are non-mechanical, we’re primarily focused on three operational areas: production management, inventory supply, and marketplaces.
Over the next few months, we’re going to be introducing the next component of our production management tool, moving from prescription to automation. We’re using machine vision to determine actual growth rates and germination rates. By feeding these rates into the production planning, growers won’t have to create growth cycles manually. The production plans will adjust based on seasonality and climate conditions on their own, with a focus on optimizing yields among crop selection. That means we can fully automate the planning component of farming.
What’s Next?
One of the biggest struggles for indoor farm operators is ensuring stable sales supply. We’re building a data set of market demand by primary buyers to help growers understand real-time demand. We’re focused on creating the marketplace for stable local purchasing.
We’re also focused on automating inventory control. Using AI, we can predict when growers are running low on various consumables and automate purchasing processes.
For more information, reach out to Allison at akopf@agrilyst.com.
The content & opinions in this article are the author’s and do not necessarily represent the views of AgriTechTomorrow
Comments (0)
This post does not have any comments. Be the first to leave a comment below.
Featured Product
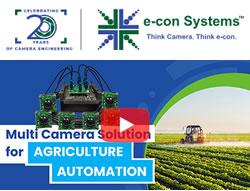